1
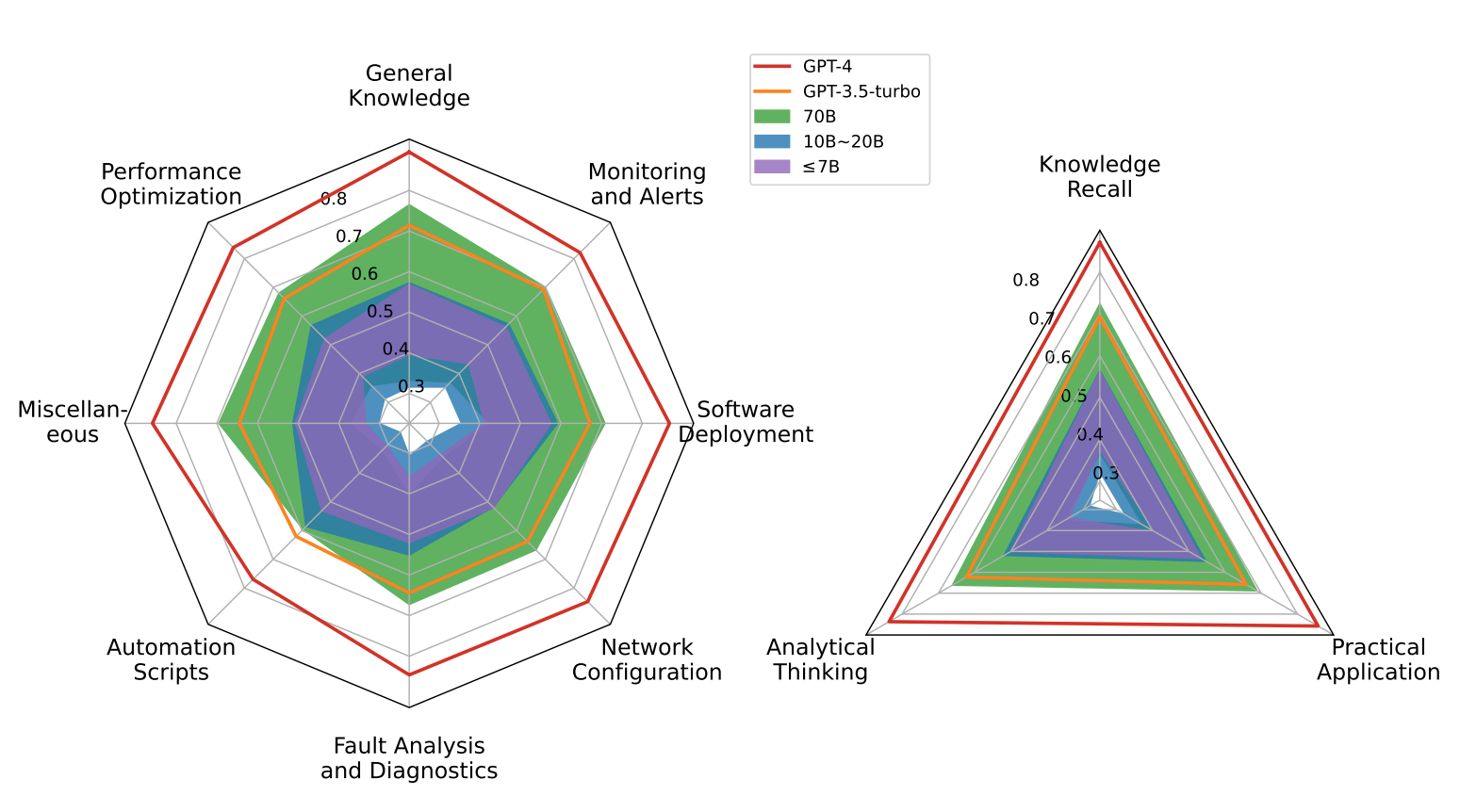
运维大模型评测榜单OpsEval
A comprehensive task-oriented AIOps benchmark designed for LLMs. For the first time, OpsEval assesses LLMs’ proficiency in three crucial scenarios (Wired Network Operation, 5G Communication Operation, and Database Operation) at various ability levels (knowledge recall, analytical thinking, and practical application). The benchmark includes 7,200 questions in both multiple-choice and question-answer (QA) formats, available in English and Chinese. With quantitative and qualitative results, we show how various LLM tricks can affect the performance of AIOps, including zero-shot, chain-of-thought, and few-shot in-context learning.
2
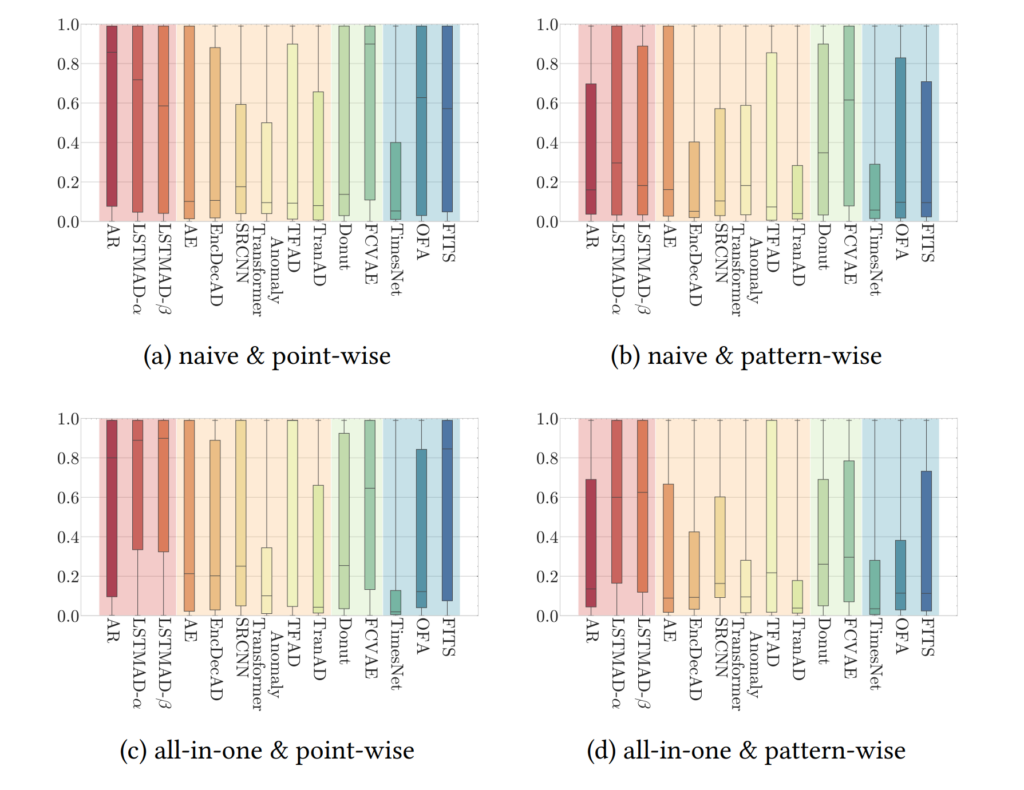
时序异常检测评测榜单TimeSeriesBench
Time series anomaly detection (TSAD) has attracted considerable scholarly and industrial interest. However, existing algorithms exhibit a gap in terms of training paradigm, online detection paradigm, and evaluation criteria when compared to the actual needs of real-world industrial systems. We propose TimeSeriesBench, an industrial-grade benchmark that we continuously maintain as a leaderboard. On this leaderboard, we assess the performance of existing algorithms across more than 168 evaluation settings combining different training and testing paradigms, evaluation metrics and datasets.
3
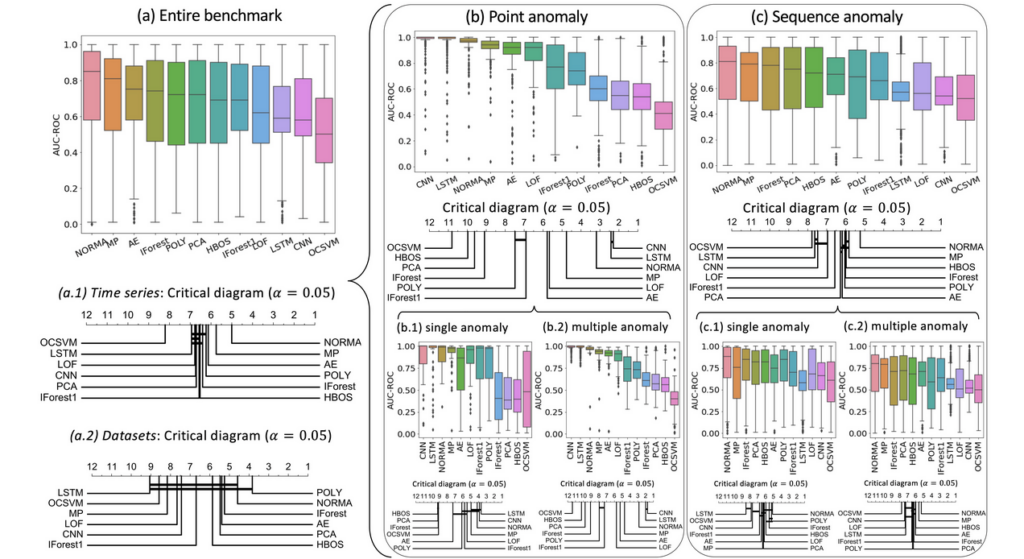
单指标时序异常检测-TSB-UAD
TSB-UAD is a new open, end-to-end benchmark suite to ease the evaluation of univariate time-series anomaly detection methods. Overall, TSB-UAD contains 12686 time series with labeled anomalies spanning different domains with high variability of anomaly types, ratios, and sizes. Specifically, TSB-UAD includes 18 previously proposed datasets containing 1980 time series from real-world data science applications.
4
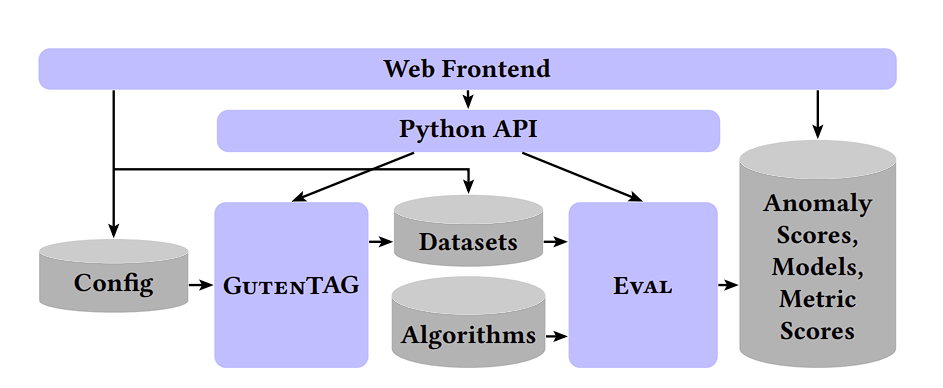
综合异常检测平台-TimeEval
TimeEval is an evaluation tool for time series anomaly detection algorithms. It defines common interfaces for datasets and algorithms to allow the efficient comparison of the algorithms’ quality and runtime performance. All algorithms are provided via docker format for easy experiments. TimeEval can be configured using a simple Python API and comes with a large collection of compatible datasets and algorithms.
5
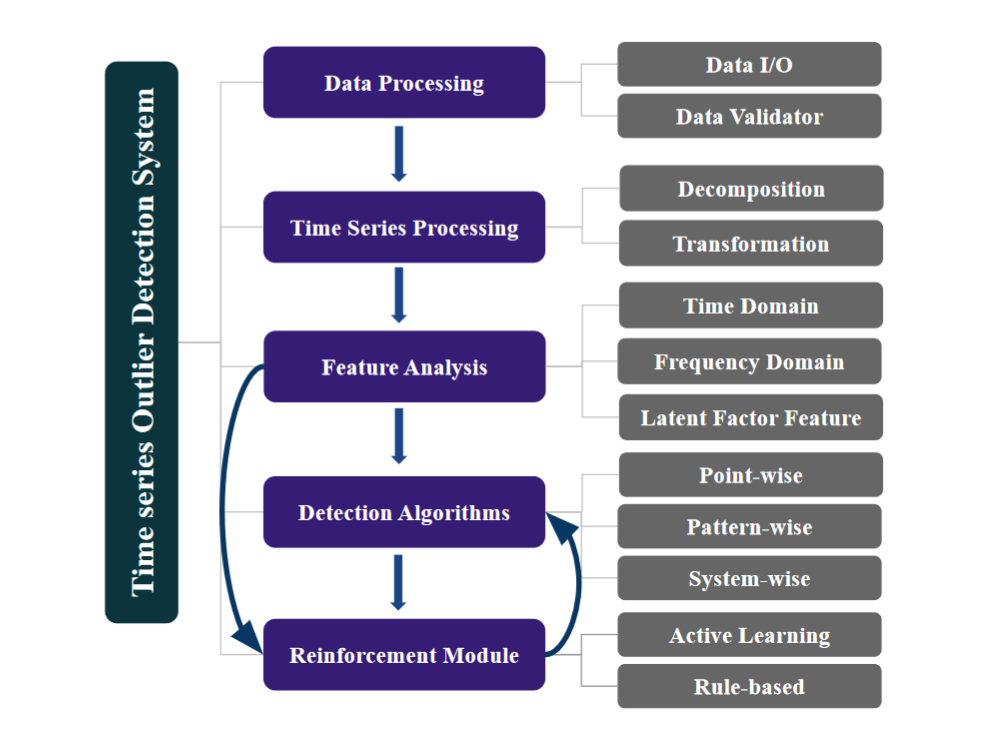
自动化时间序列异常检测系统-TODS
TODS is a full-stack automated machine learning system for outlier detection on multivariate time-series data. TODS provides exhaustive modules for building machine learning-based outlier detection systems, including: data processing, time series processing, feature analysis (extraction), detection algorithms, and reinforcement module. The functionalities provided via these modules include data preprocessing for general purposes, time series data smoothing/transformation, extracting features from time/frequency domains, various detection algorithms, and involving human expertise to calibrate the system.